THE PROBLEM
Management of air traffic during the arrival phase in the Terminal Maneuvering Area (TMA) is becoming increasingly complex and can result in very high workloads for Air Traffic Controllers (ATCO), especially when traffic loads become high/very high. This may lead to a loss of efficiency in the management of arrival trajectories, in particular when delivering aircraft to intercept the final approach path / localiser with optimal spacing. Consequently, this may impact airport capacity, flight path efficiency, runway throughput, and operational safety during these periods.
As a result, additional capacity issues can propagate across the wider Air Traffic Management (ATM) network, particularly if several airports experience this type of issue at the same time. Moreover, as new airspace users with different performance characteristics to conventional aircraft are integrated into the airport environment, additional capacity/efficiency challenges may manifest themselves, rendering the ATCO task even more difficult and complex.
THE SOLUTION
The ORCI research will investigate a new Artificial Intelligence (AI) based Decision Support Tool (DST) that can help to enhance ATCO performance in the TMA approach phase, even as traffic demand and complexity becomes very high.
The objective is to provide key information to Air Traffic Controllers in final approach sectors, to support informed decisions on when to issue vectoring instructions to aircraft for optimal spacing between consecutive arrivals.
“What distance between consecutive arrivals would be achieved at the ILS localiser interception point if the ATCO started issuing vectoring instructions right now?”
This is particularly important given the increasing pressure on ATCO communications, where higher traffic levels and operational demands are leading to communication overload. By reducing the need for excessive vectoring communications, ORCI aims to lower the workload on ATCOs, enabling them to manage traffic more efficiently, and focus on critical decision-making, ultimately contributing to an optimal arrival stream at the airport.
HOW ARE WE DOING THIS?
We follow a structured and iterative process to develop our Machine Learning model, ensuring it meets operational needs and delivers reliable results. It all starts with defining the problem, where we collect and process raw data, clean it, and parameterize use cases. Next comes model development, where we select, optimize, and train the model. Once trained, it goes through evaluation—if it doesn’t meet expectations, we refine and retrain it; if successful, we proceed to validation. In this phase, we create real-world scenarios and conduct validation exercises to assess its effectiveness. The final step is analyzing results to ensure continuous improvement and alignment with our objectives.
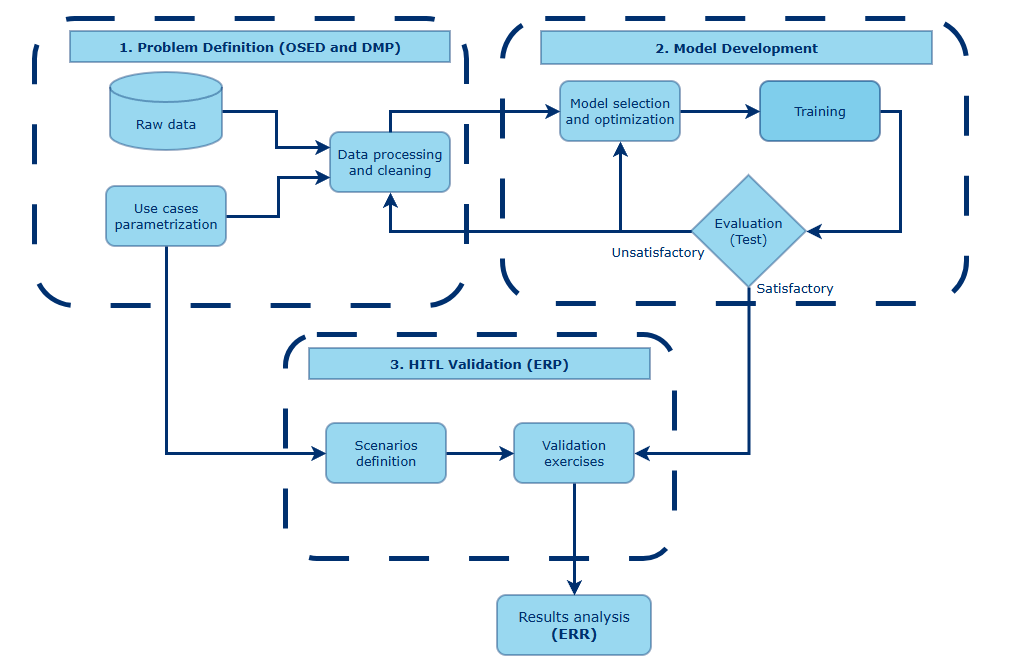
USE CASES
BARCELONA
Type of operations:
• Trombone-shape RNAV procedure
• RWY 24R (WRL parallel runway configuration – segregated mode)
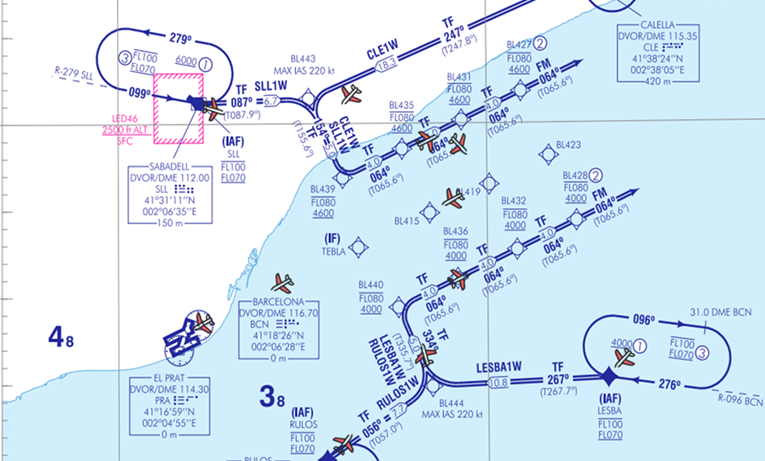
Day to day operations (RWY 24R):
• The aircraft fly along the outbound leg until the ATCo allows it to perfom a 180 degrees turn to intercept Localiser and fly towards TEBLA (IF).
• ORCI is expected to be used, mainly but not exclusively, in high/very high demand periods (Trombone use).
• The greater the demand pressure, the longer the flown segments in the trombone are.
• Once established in LOC, distances between aircrafts cannot be significantly altered (Speed control).
LISBON
Type of operations:
• Point-Merge System (PMS)
• RWY 02 (ARR/DEP – mixed mode)
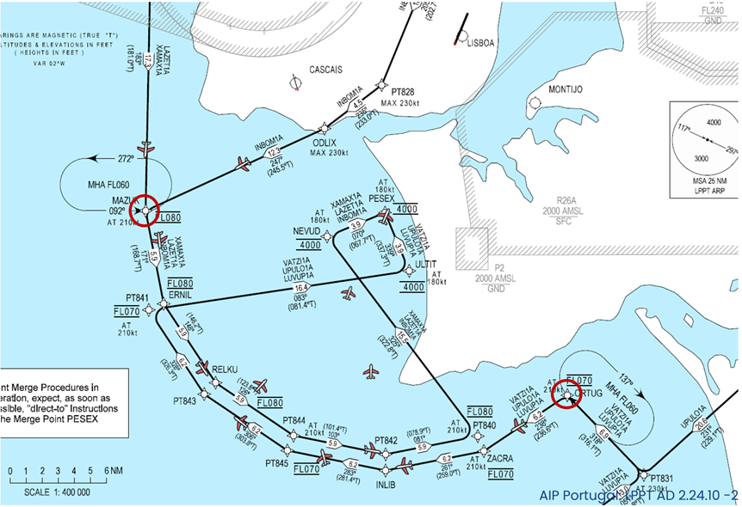
Day to day operations (RWY 02):
• The aircraft fly along the PMS arc until the ATCo allows it to perfom a 90 degrees turn towards PESEX (IF).
• ORCI is expected to be used, mainly but not exclusively, in high/very high demand periods (PMS use).
• The greater the demand pressure, the longer the flown arc segments in the PMS are.
• Once established in LOC, distances between aircrafts cannot be significantly altered (Speed control).